Introduction
Data mining is a term that has been thrown around a lot in recent years. While it sounds like a simple concept, but there is much more to it than meets the eye. In this article, we will take a deep dive into what data mining is and how it can benefit your business.
Definition
Data mining is the process of analyzing large sets of data to discover patterns, correlations, and other useful information. It involves using various statistical and computational techniques to extract valuable insights from data that might not be apparent otherwise.
Importance of Data Mining for businesses
It can provide businesses with a competitive edge by helping them make more informed decisions. By identifying patterns and trends in customer behavior, businesses can gain insights into what their customers want and how to better serve them.
Overview of the Benefits of data mining
The benefits of mining data are multifold, ranging from improved customer satisfaction to increased profits. Some of the key benefits of mining for businesses include:
- Improved decision-making
- Better customer insights
- Increased revenue
- Improved efficiency and productivity
- More accurate forecasting
Understanding Data Mining
The Concept
Data mining is a complex process that involves several steps, including data collection, preprocessing, modeling, and evaluation. The ultimate goal is to discover useful insights that can help businesses make better decisions.
The Role of data mining in business decision-making
Data mining plays a crucial role in business decision-making by providing valuable insights into customer behavior, market trends, and other key factors that affect business performance. By using data mining techniques, businesses can make more informed decisions that are based on data rather than intuition.
How data mining is different from other data analysis techniques
Data mining is different from other data analysis techniques, such as data warehousing and business intelligence, in that it focuses on discovering patterns and insights that might not be apparent through other methods. It involves a more exploratory approach to data analysis, with an emphasis on discovering new insights and relationships in data.
Applications of Data Mining in Business
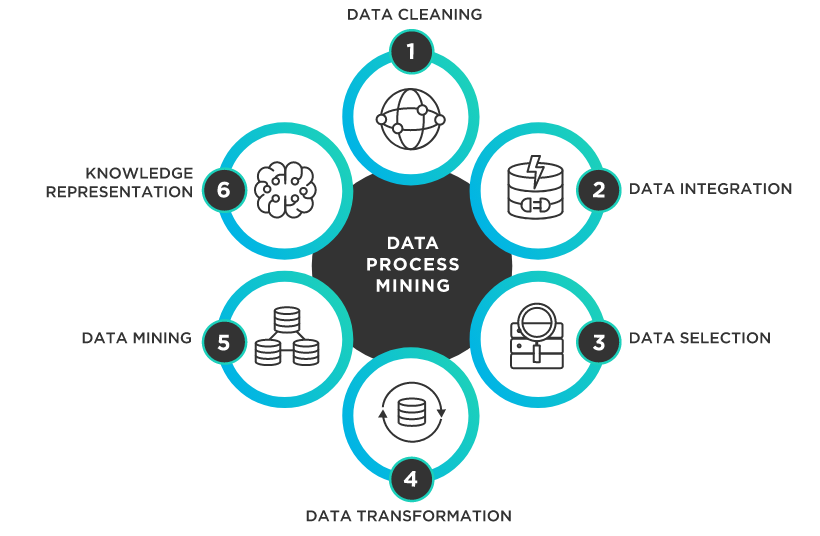
1. Customer profiling and segmentation
It can be used to create customer profiles and segments based on their behavior, preferences, and other factors. By understanding their customers better, businesses can tailor their products and services to meet their needs and improve customer satisfaction.
2. Predictive analytics for sales forecasting
Predictive analytics involves using historical data to forecast future trends and behavior. This can be especially useful for sales forecasting, allowing businesses to anticipate demand and adjust their production and marketing accordingly.
3. Fraud detection and prevention
It can be used to detect and prevent fraud by identifying patterns and anomalies in data that may indicate fraudulent behavior. This can help businesses save money and protect their reputation.
4. Sentiment analysis for customer feedback
Sentiment analysis involves analyzing customer feedback to determine their attitudes and opinions about a product or service. This can help businesses improve their products and services based on customer feedback.
5. Supply chain optimization
Supply chain optimization involves identifying inefficiencies and bottlenecks in the supply chain by using data. By streamlining the supply chain, businesses can reduce costs and improve efficiency.
The Data Mining Process
The Mining process typically involves the following steps:
Data collection and preparation: This involves gathering relevant data from various sources and preparing it for analysis. This may involve cleaning and formatting the data to ensure that it is accurate and consistent.
Exploratory data analysis: This involves using statistical techniques and visualization tools to explore the data and identify patterns and relationships. This may involve identifying outliers, trends, and correlations in the data.
Model building and testing: This involves developing models based on the data to make predictions or identify patterns. This may involve using statistical models, machine learning algorithms, or other techniques.
Evaluation and interpretation of results: This involves evaluating the performance of the models and interpreting the results. This may involve assessing the accuracy and reliability of the models and determining the significance of the results.

Tools and Techniques
There are many tools and techniques available for mining data effectively. Here are some of the most common:
Statistical analysis tools: These tools are used to analyze data using statistical techniques such as regression analysis, hypothesis testing, and ANOVA. They are particularly useful for analyzing numerical data.
Machine learning algorithms: These algorithms are used to build predictive models based on data. They can be used to identify patterns and relationships in data and make predictions about future events.
Text mining and natural language processing: These techniques are used to analyze unstructured data such as text documents, social media posts, and emails. They can be used to extract meaningful information from large volumes of text data.
Visualization tools: Tools such as Tableau, Power BI, Microsoft Excel, etc. are used to create visual representations of data, such as graphs and charts. They can be used to identify patterns and trends in data and communicate insights to stakeholders.
Challenges and Limitations
Despite its many benefits, data mining does come with a few challenges and limitations that businesses need to be aware of. Here are some of the main issues to consider:
- Data quality and accuracy: One of the biggest challenges with mining is ensuring the accuracy and completeness of the data being used. Data that is incomplete or inaccurate can lead to flawed insights and decisions.
- Privacy and ethical concerns: With the increasing amount of data being collected and analyzed, privacy and ethical concerns have become a major issue in the field. Businesses must ensure that they are using data in a responsible and ethical manner, and take steps to protect customer privacy.
- Interpretation of results and bias: It is not a perfect science, and the results generated by the algorithms can be subject to interpretation and bias. It’s important to carefully evaluate and interpret the results of such algorithms to ensure that they are accurate and unbiased.
- Implementation and maintenance costs: Mining requires significant resources, including skilled staff, hardware and software, and data storage and processing capabilities. These costs can be a significant barrier for small and medium-sized businesses.
Want to stay Updated on the Latest Trends in Data Science
Like what you’re reading? Share it now:
Best Practices for Successful Data Mining
To ensure that your mining is successful and delivers meaningful insights, businesses should follow these best practices:
- Defining business goals and objectives: Before embarking on a mining project, businesses should clearly define their goals and objectives. This will help ensure that the mining effort is focused and relevant.
- Choosing the right data sources: The quality and relevance of the data used in mining is critical. Businesses should carefully choose the data sources that will be used for analysis, and ensure that the data is accurate, relevant, and complete.
- Selecting appropriate mining techniques: There are many different techniques available, and businesses should choose the one that is most appropriate for their needs. This may involve using a combination of techniques to achieve the desired results.
- Collaborating with data scientists and experts: Mining is a complex process, and businesses should work with experienced data scientists and experts to ensure that the process is carried out effectively.
- Monitoring and evaluating the results: Mining is an ongoing process, and businesses should continuously monitor and evaluate the results to ensure that they are achieving their goals and objectives.
Conclusion
In conclusion, data mining is a powerful tool that can provide valuable insights and help businesses make better decisions. By understanding the concept, its applications, the process, the tools and techniques involved, and the challenges and limitations that come with it, businesses can develop a successful mining strategy that delivers meaningful results. As the Industry continues to evolve and become more sophisticated, it will become an increasingly important tool for businesses in a wide range of industries.
FAQs
What types of data can be mined?
Mining can be applied to various types of data including structured data, unstructured data, and semi-structured data.
How can data mining help with customer profiling and segmentation?
Mining techniques can analyze customer data to identify patterns and characteristics that can be used to group customers into segments based on demographics, behavior, preferences, etc.
Can mining be used to prevent fraud in business?
Yes, Mining techniques can be used to detect and prevent fraudulent activities by analyzing patterns and anomalies in transactional data.
What is the difference between data mining and data analysis?
Data mining is a subset of data analysis that focuses on discovering patterns and relationships in large datasets using machine learning and statistical algorithms.
How can businesses ensure the success of their mining projects?
To ensure the success of a mining project, businesses should define clear goals and objectives, select the right data sources and mining techniques, collaborate with experts, and continuously monitor and evaluate the results.
1 Comment
Piyush MK
Hello Elementor